What if you knew just how much to sanitize (legally protected) data in order to make it freely shareable?
Sharing data in the twenty-first century is fraught with error. Most com-
monly, data is freely accessible, surreptitiously stolen, and easily capitalized in the pur-
suit of monetary maximization. But when data does find itself shrouded behind the veil
of “personally identifiable information” (PII), it becomes nearly sacrosanct, impenetrable
without consideration of ambiguous (yet penalty-rich) statutory law—inhibiting utility.
Either choice, unnecessarily stifling innovation or indiscriminately pilfering privacy,
leaves much to be desired.
This Article proposes a novel, two-step test for creating futureproof, bright-line
rules around the sharing of legally protected data. The crux of the test centers on identi-
fying a legal comparator between a particular data sanitization standard—differential pri-
vacy: a means of analyzing mechanisms that manipulate, and therefore sanitize, data—
and statutory law. Step one identifies a proxy value for reidentification risk which may
be easy calculated from an ε-differentially private mechanism: the guess difference. Step
two finds a corollary in statutory law: the maximum reidentification risk a statute toler-
ates when permitting confidential data sharing. If step one is lower than or equal to step
two, any output derived using the mechanism may be considered legally shareable; the
mechanism itself may be deemed (statute, ε)-differentially private.
The two-step test provides clarity to data stewards hosting legally or possibly legally
protected data, greasing the wheels in advancements in science and technology by
providing an avenue for protected, compliant, and useful data sharing.
We teach differential privacy using simple, easy-to-grasp concepts.
Like the difference between these two images. One without data sanitization (left) and one with privacy-preserving redactions (right). We can still see that this is a bike on the right, but we deleted with 50% probability every single charcter in the image.
We present an easy-to-apply test for assessing the legal compliance of a privacy-preserving mechanism—the risk of reidentification a mechanism provides.
We need futureproof, bright-line rules around the sharing of legally (or potentially legally) protected data. Here, we introduce a two-step test that allows any differentially private mechanism to be sized up against legal requirements. The test produces a clear, simple, single number as output, thereby permitting a judge, data steward, or user to assess privacy risk without getting bogged down in the details.
Guess Difference ≈ Risk of Reidentification
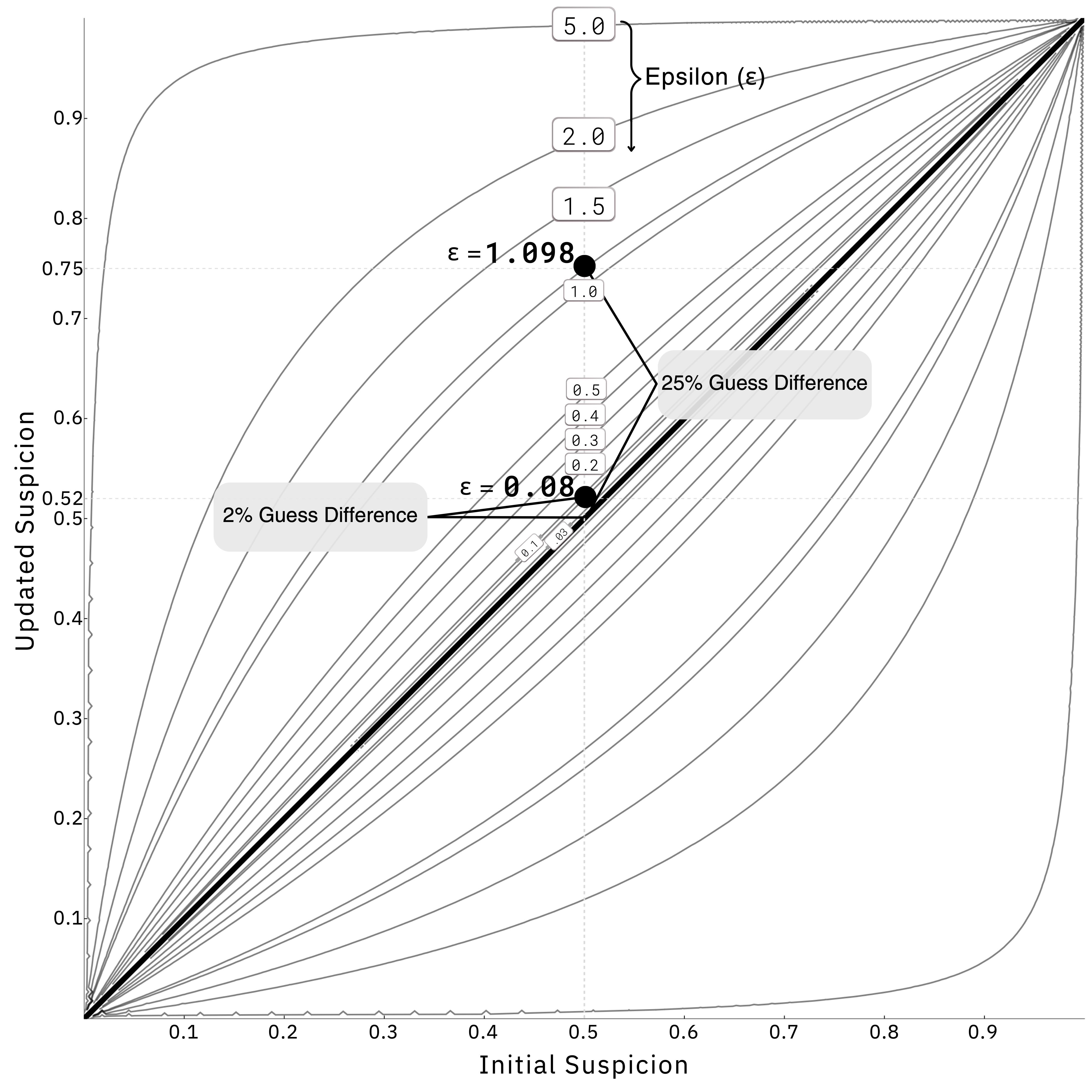